How Machine Learning is Transforming Business Decision-Making
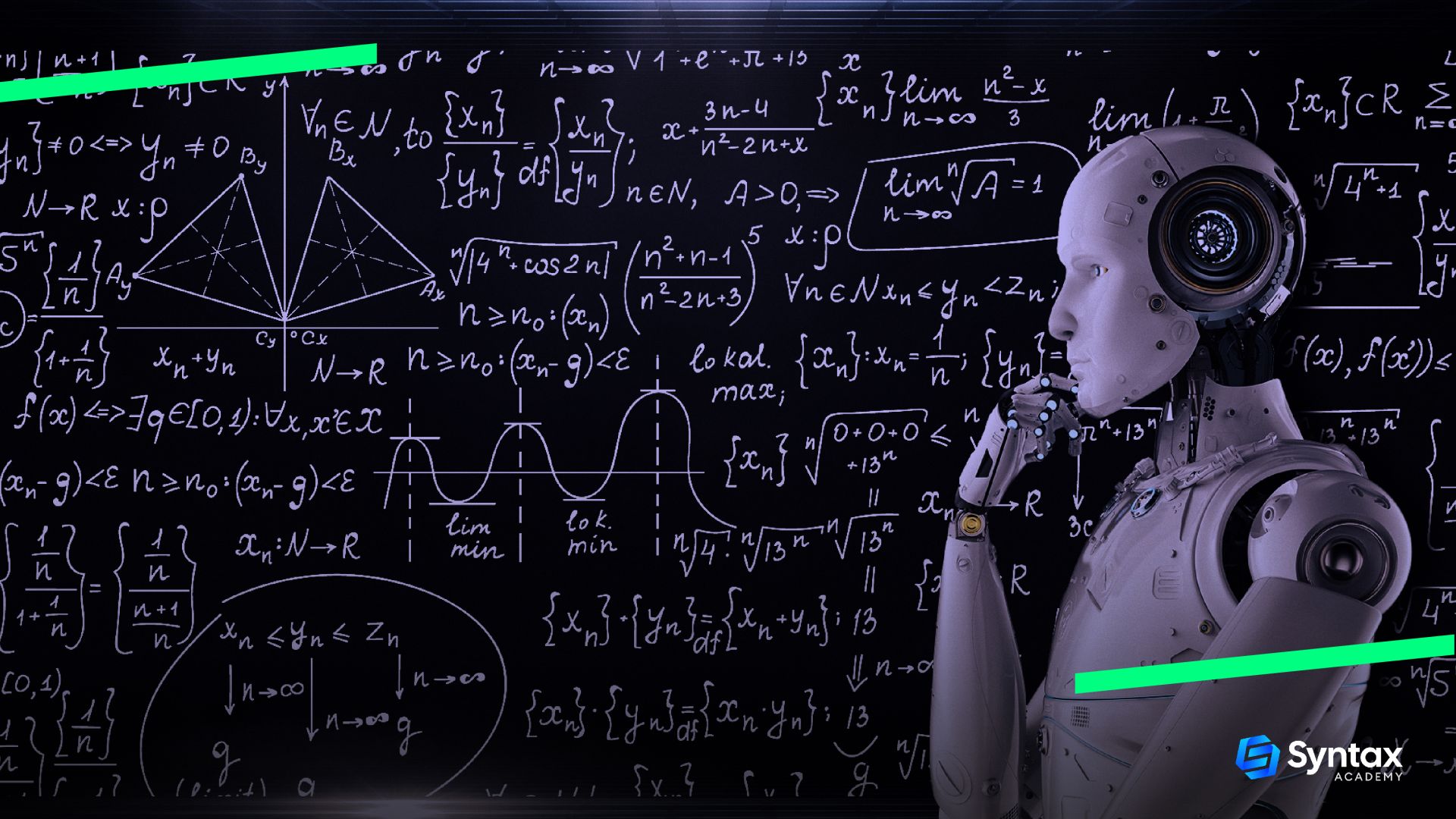
Table of Contents
-
1. What is Machine Learning?
-
4. Possible Impacts of Machine Learning on Business Decision-Making
-
2. What is Business Decision-Making?
-
5. Conclusion
-
3. How Can ML Be Integrated into Business?
1. What is Machine Learning?
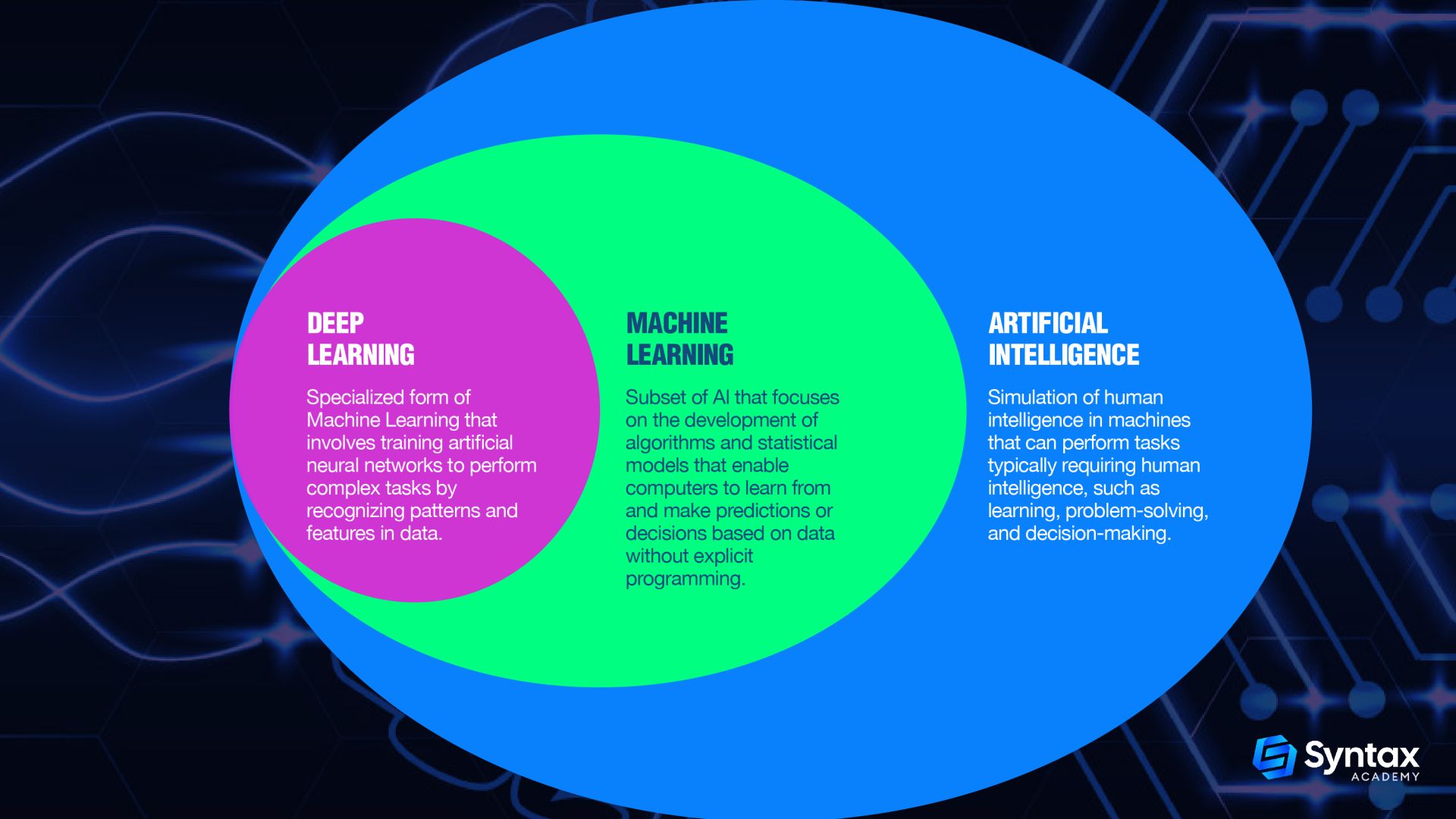
- Key Points about Machine Learning
- Data-Driven: Relies heavily on data to generate accurate predictions.
- Adaptive: Learns and improves over time with more data.
- Automation: Automates complex tasks that would be difficult for humans to perform manually.
2. What is Business Decision-Making?
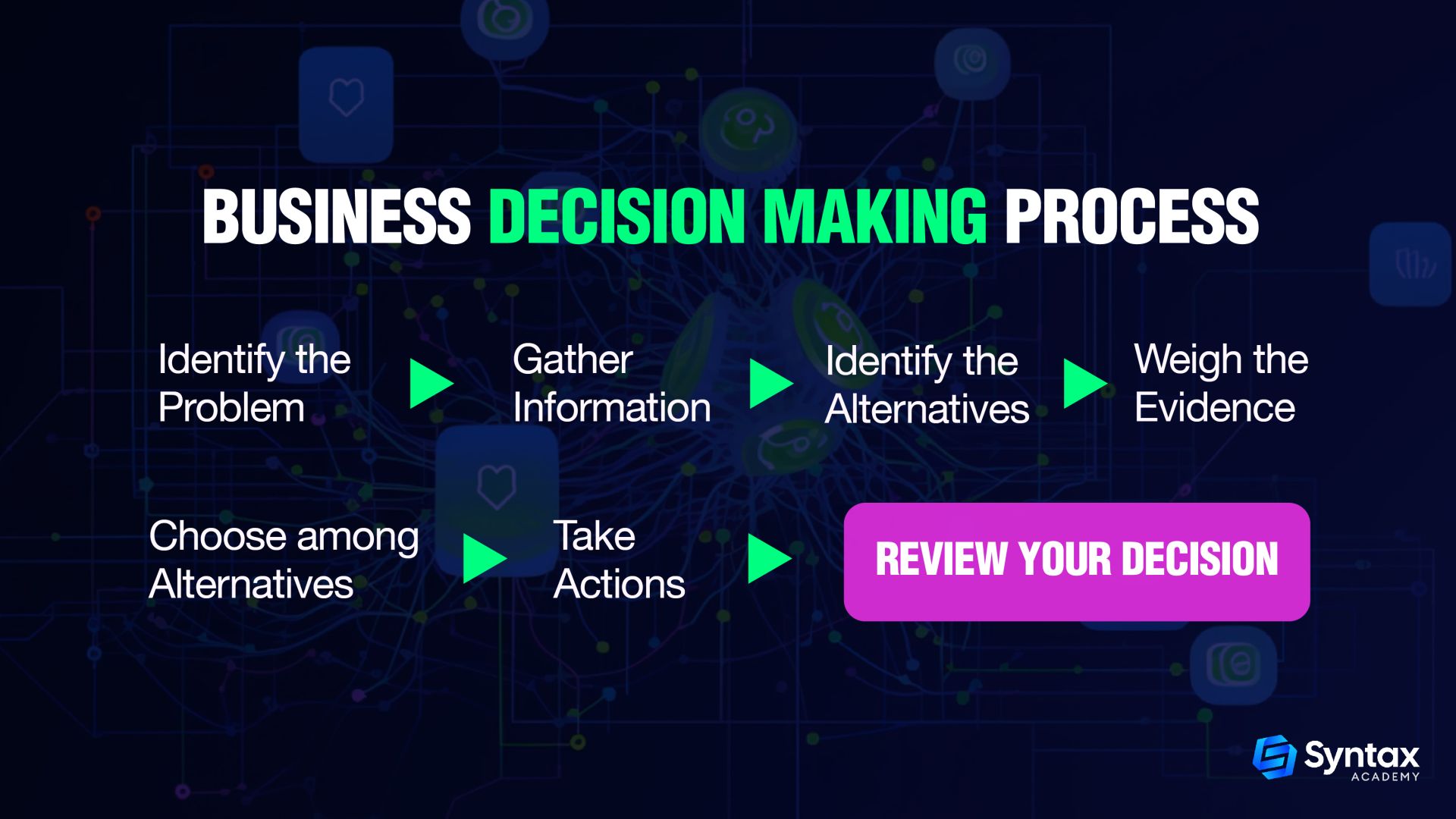
- Data Analysis: Collecting and analyzing relevant data.
- Strategic Planning: Developing long-term plans and goals.
- Risk Management: Identifying and mitigating risks.
- Resource Allocation: Efficiently using resources to achieve objectives.
3. How Can ML Be Integrated into Business?
- Identify Use Cases: Determine where ML can add value, such as in customer segmentation, demand forecasting, or fraud detection.
- Data Collection and Preparation: Gather and clean data to train ML models.
- Model Development: Develop and train ML models using historical data.
- Implementation: Deploy models into existing business systems.
- Monitoring and Maintenance: Continuously monitor model performance and update as needed.
- Example:
- A retail company might use ML to analyze customer purchase history, enabling personalized marketing and inventory management.
4. Possible Impacts of Machine Learning on Business Decision-Making
- Data-Driven Decisions:
- ML algorithms can process vast amounts of data quickly and accurately, identifying patterns and correlations that might be missed by human analysis.
- This leads to more informed and precise decision-making.
- Example:
In finance, ML models can analyze market trends and predict stock prices, helping traders make better investment decisions.
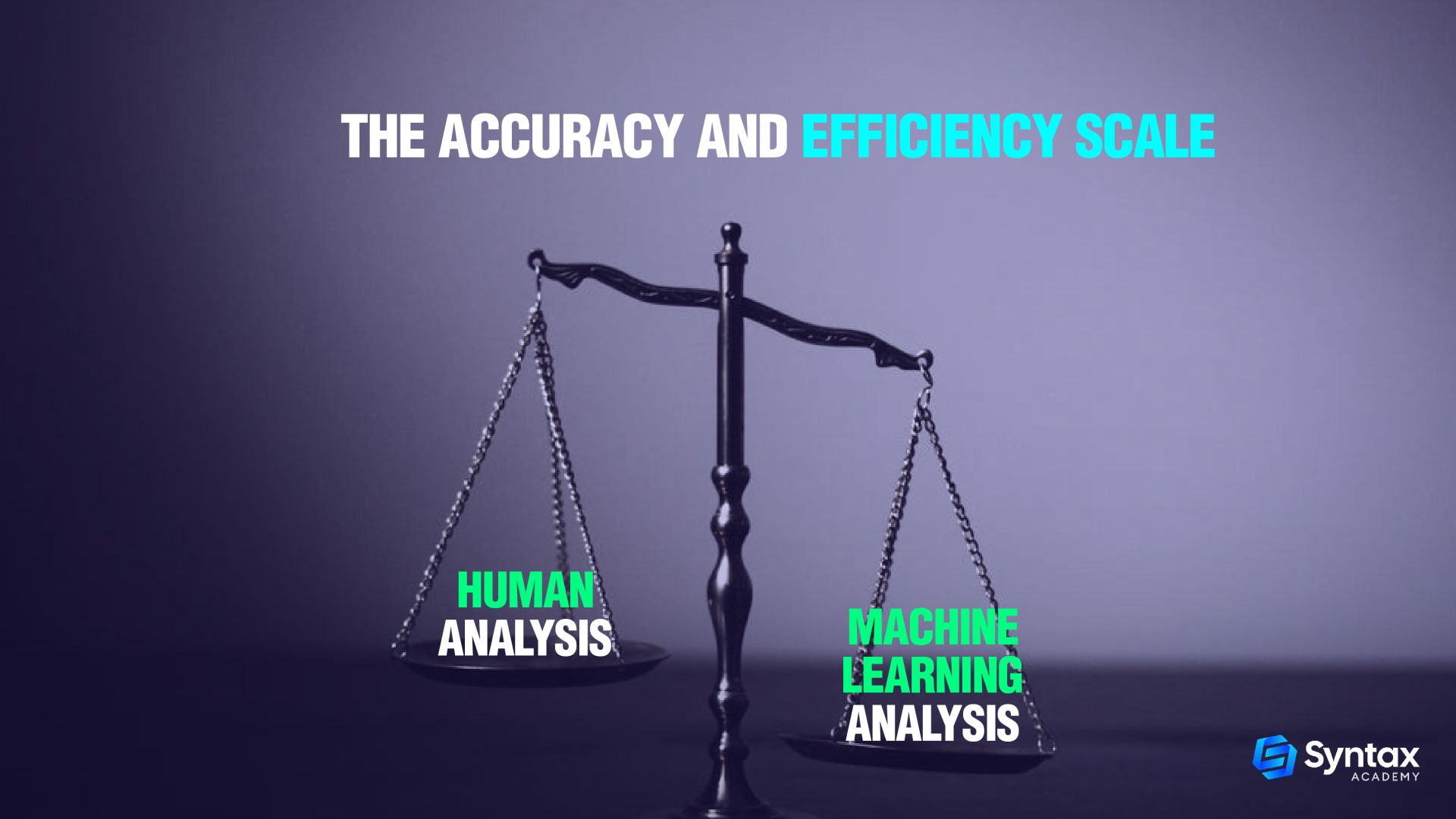
- ML models can forecast future trends and behaviors based on historical data.
- Example:
A company can use predictive analytics to anticipate customer demand, adjust inventory levels, and optimize supply chain management.
- Personalization:
- ML can analyze customer data to provide personalized experiences, improving customer satisfaction and loyalty.
- Example:
Streaming services like Netflix use ML to recommend content tailored to individual user preferences, increasing user engagement and retention.
- Segmentation:
- Businesses can use ML to identify distinct customer segments and tailor their marketing efforts to each group.
- Example:
An e-commerce platform might segment customers based on their browsing and purchase history, sending targeted promotions that are more likely to convert.
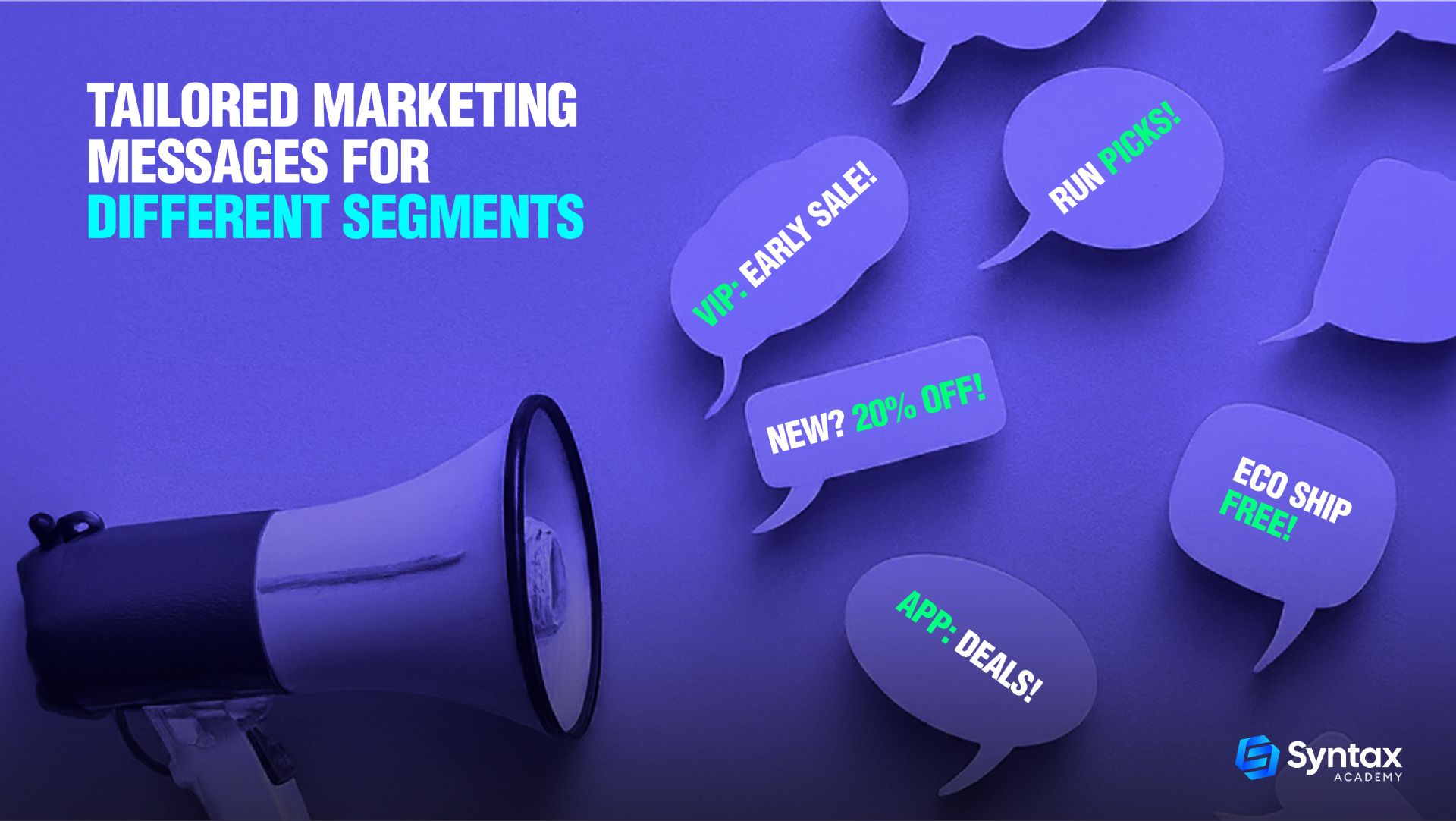
- Cost Reduction:
- Operational Efficiency
- Automating routine tasks with ML reduces operational costs and frees up human resources for more strategic activities.
- Example:
ML-powered chatbots can handle customer service inquiries, allowing human agents to focus on more complex issues.
- Resource Optimization:
- ML helps optimize resource allocation by predicting the most efficient use of resources.
- Example:
ML models can forecast the best times to run marketing campaigns based on customer behavior, maximizing the return on investment.
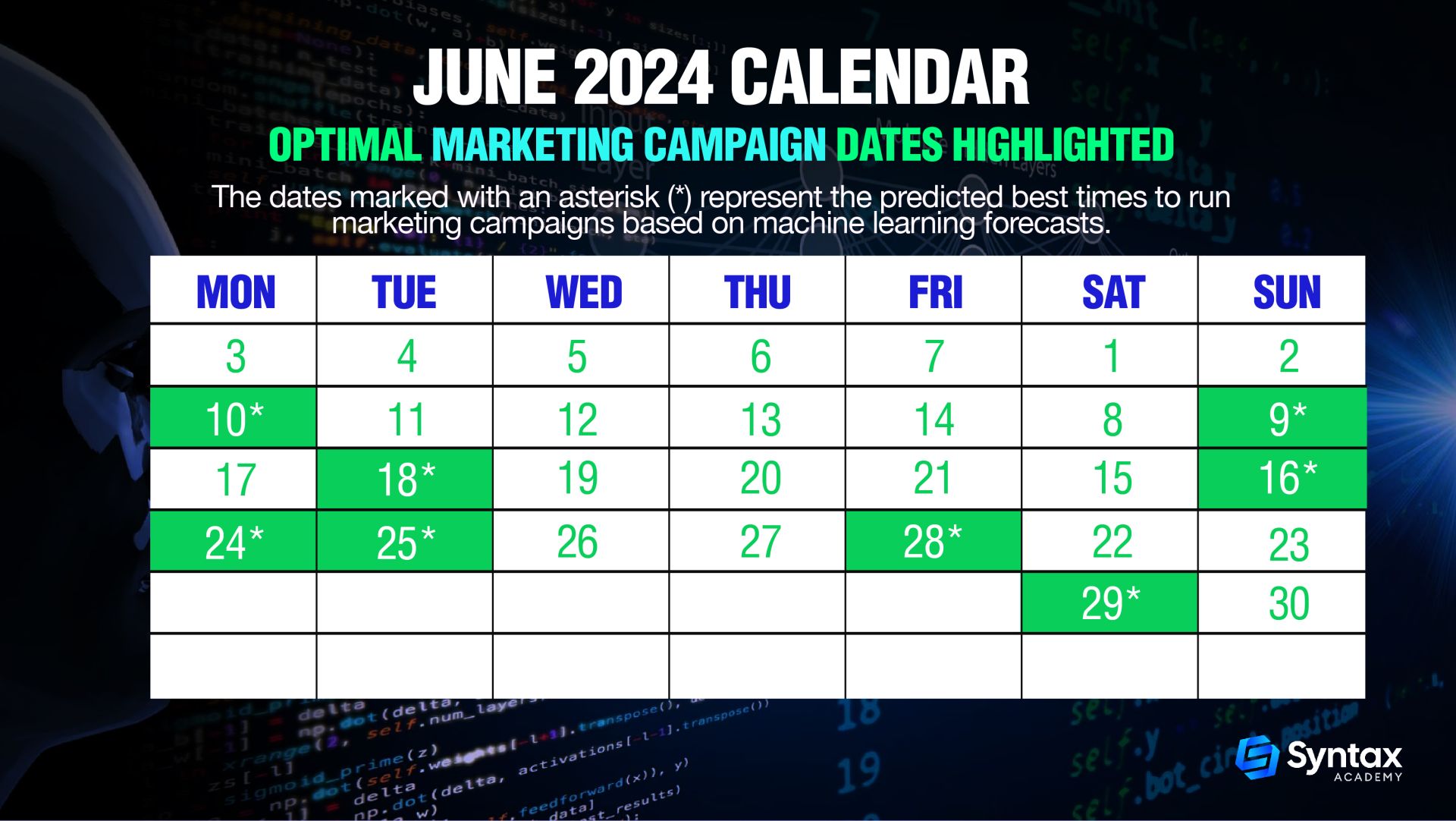
- Risk Management
- Fraud Detection:
- ML algorithms can detect fraudulent activities by analyzing transaction patterns and identifying anomalies.
- Example:
Banks use ML to monitor transactions in real-time, flagging suspicious activities and preventing fraud before it causes significant damage.
- Credit Scoring:
- ML models assess credit risk more accurately than traditional methods by considering a wider range of data points.
- This helps financial institutions make better lending decisions, reducing the risk of defaults.
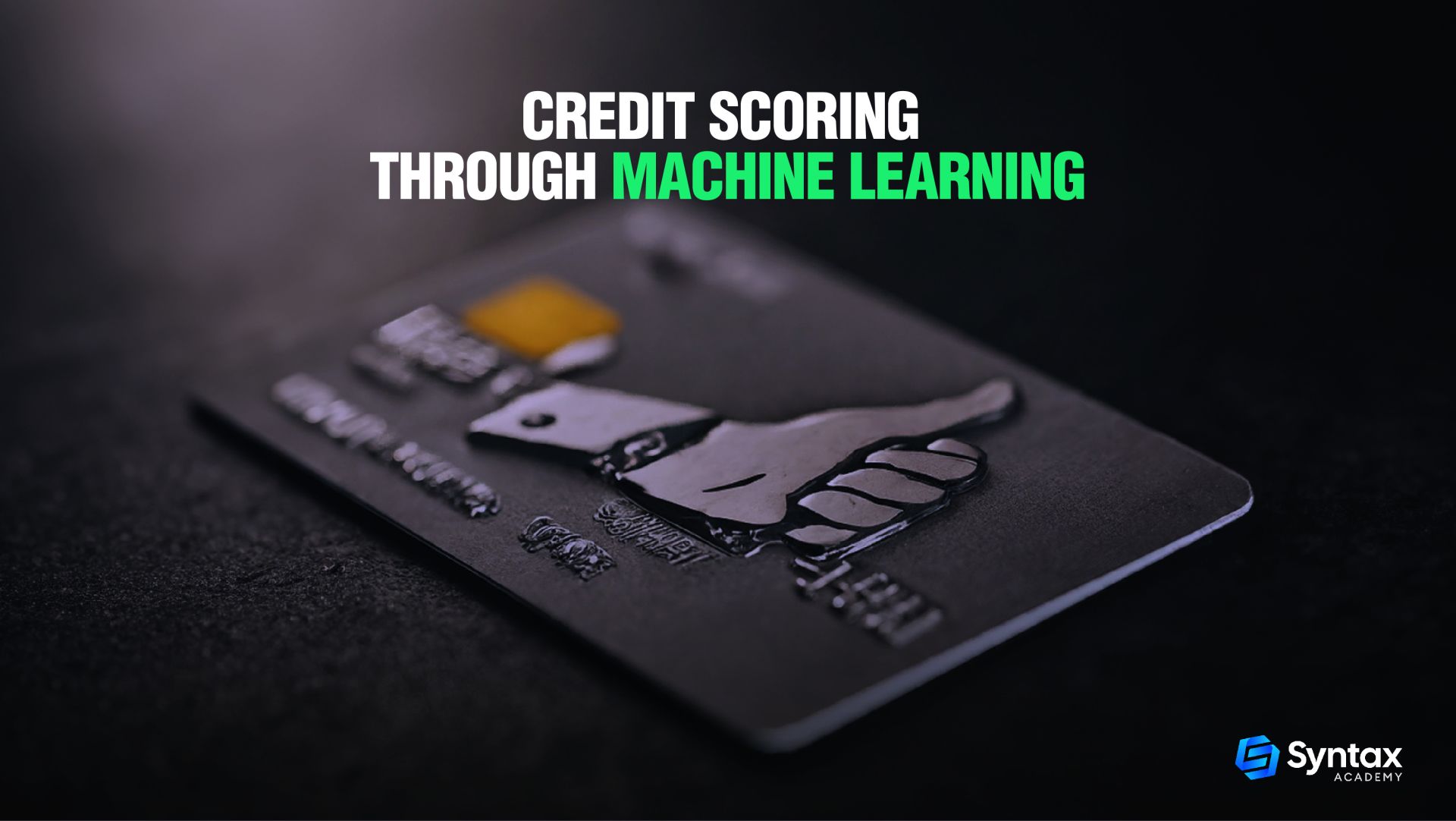
- Market Analysis:
- ML can analyze market data to identify emerging trends and competitive threats, providing businesses with actionable insights to stay ahead of the competition.
- Example:
Retailers can use ML to analyze competitor pricing strategies and adjust their prices dynamically.
- Product Innovation:
- By analyzing customer feedback and market trends, ML can help businesses identify opportunities for new product development.
- Example:
Consumer goods companies can use ML to analyze social media data and identify unmet customer needs, driving innovation and growth.
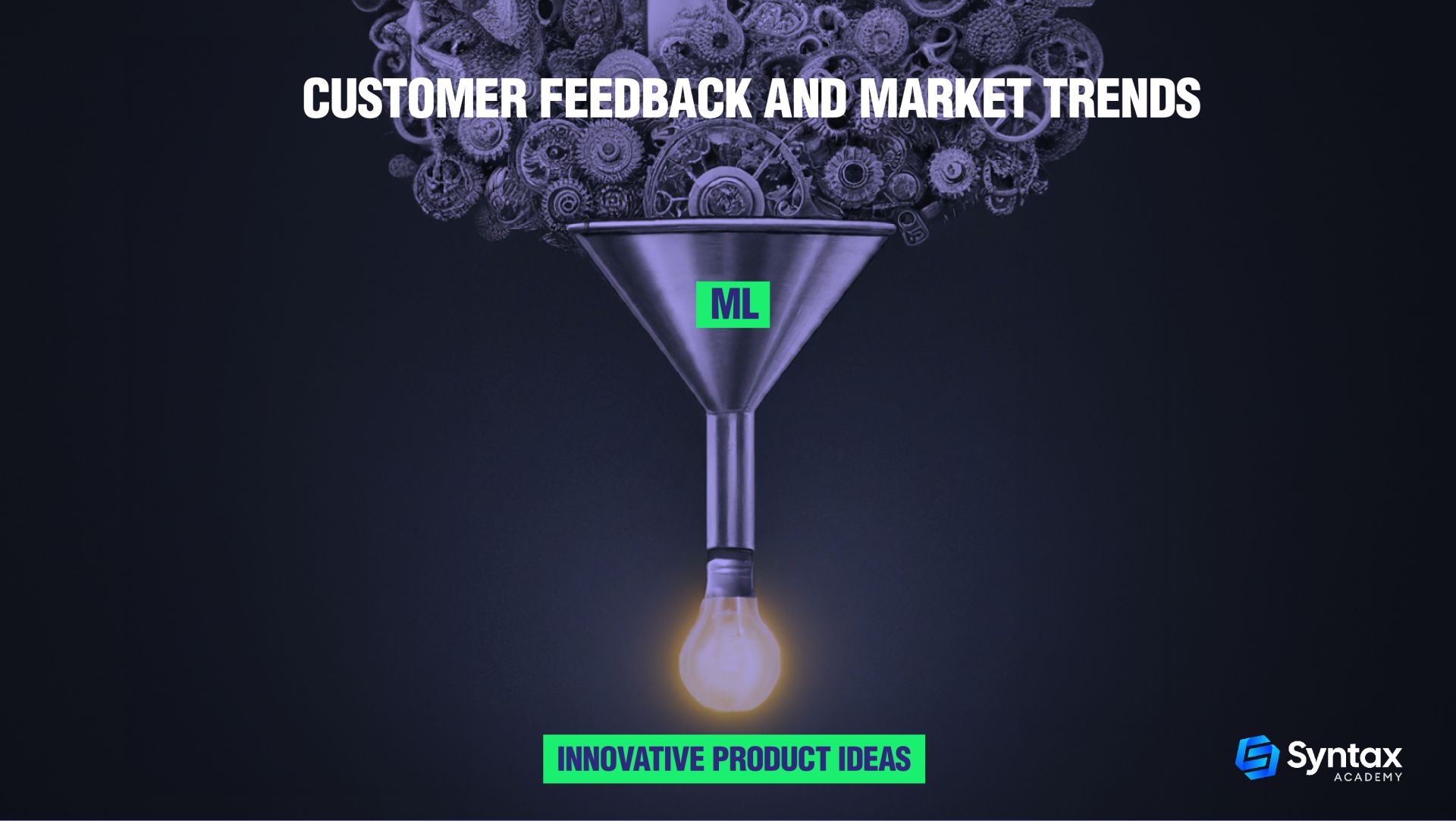
Data Quality and Quantity
- Data Dependency:
- ML models need large, high-quality datasets. Incomplete or biased data can lead to inaccurate predictions.
- Example:
In healthcare, biased patient data might cause misdiagnoses.
- Data Privacy:
- Protecting sensitive data requires strict privacy measures.
- Example:
Financial institutions must comply with data privacy regulations like GDPR.
- Complexity and Cost
- Implementation Costs:
- Developing ML models can be expensive and time-consuming.
- Example:
Setting up ML infrastructure can be costly for small businesses.
- Skilled Workforce:
- Specialized skills are needed to develop and maintain ML models.
- Example:
Hiring data scientists and ML engineers can be competitive and expensive.
- Technical Challenges
- Model Interpretability:
- Many ML models are "black boxes" with decision processes that are hard to interpret.
- Example:
Explaining a credit decision made by a neural network can be difficult.
- Scaling ML solutions can require extensive computing resources.
- Example:
Retailers may struggle to scale recommendation systems during peak seasons.
5. Conclusion
Machine Learning is transforming business decision-making by improving accuracy, efficiency, and insights. Despite challenges, such as data quality and costs, ML offers significant benefits that help businesses make informed decisions, predict trends, and personalize customer experiences. Embracing ML will continue to drive innovation and maintain competitiveness in a data-driven future. Interested in mastering ML? Explore Artificial Intelligence/Machine Learning courses at Syntax Academy to gain hands-on skills in data analysis, model development, and implementation. Equip yourself with the tools needed to leverage ML effectively and propel your career in the tech-driven world of tomorrow.
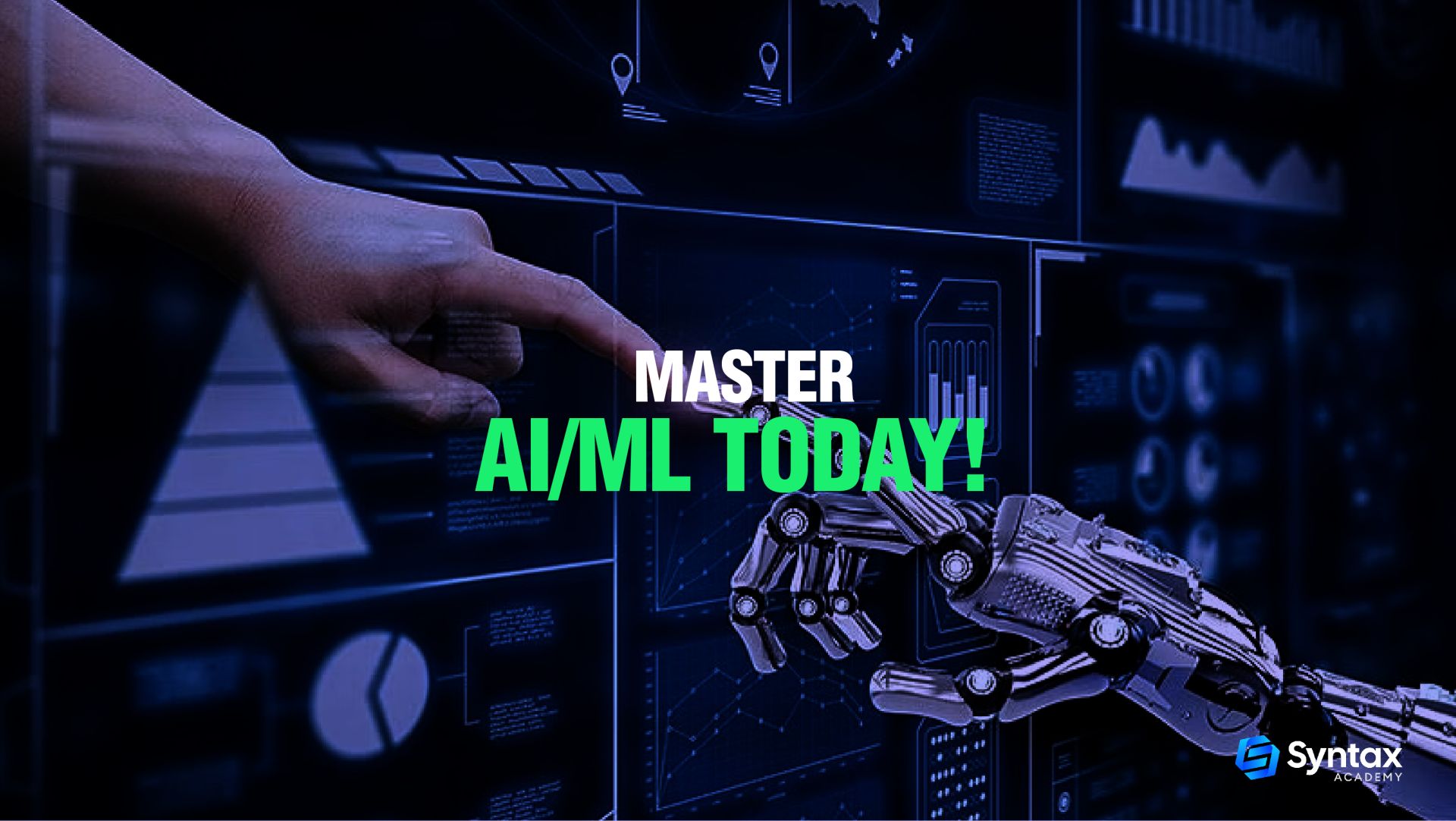
Share with your community!
Related Article
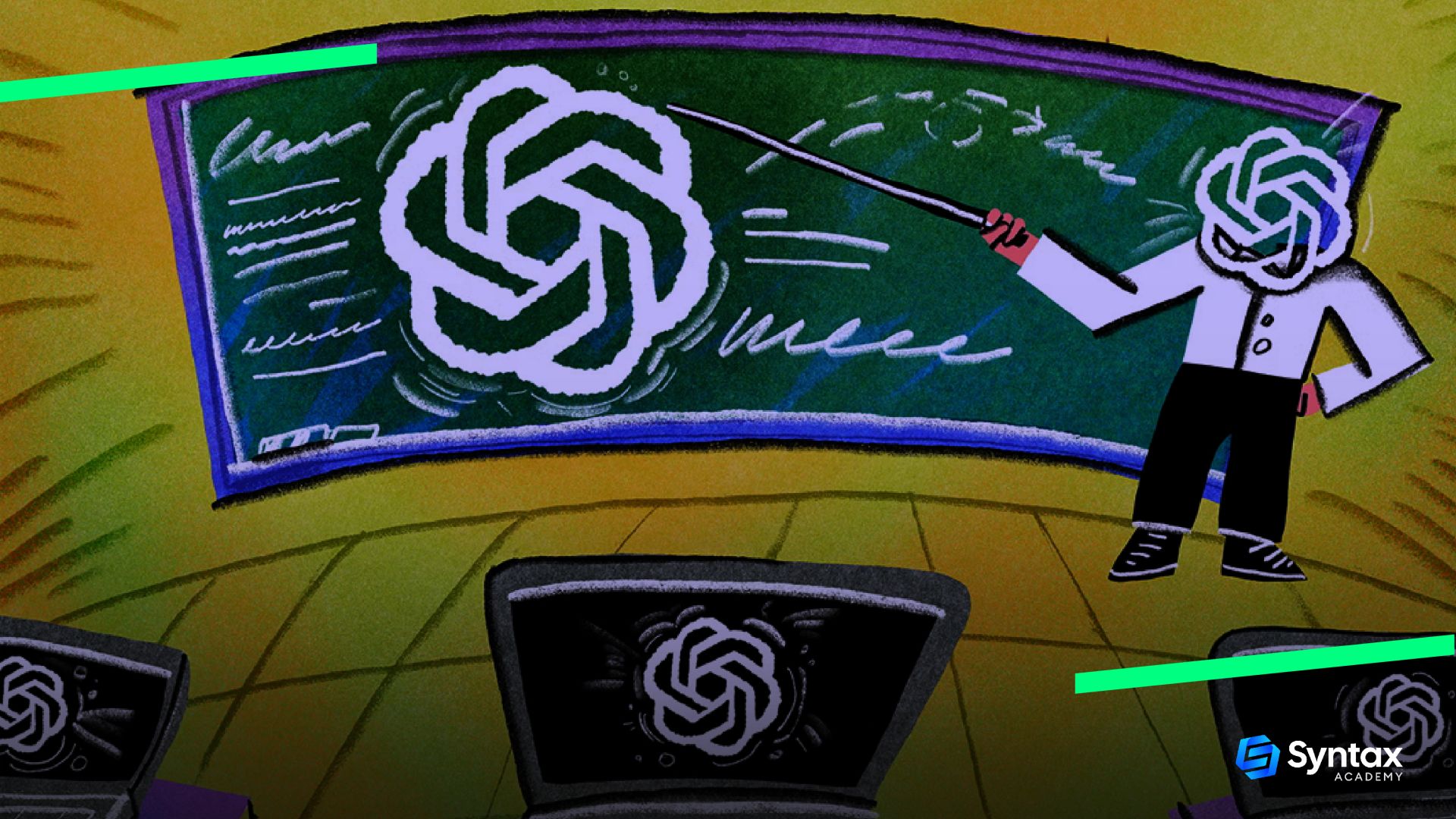
ChatGPT
Mastering ChatGPT: A Comprehensive Guide to
Getting Started
By: Martha James
Imagine having a tool that can chat with you, help you write, debug your code, and even spark creative ideas—all in one place. That’s exactly what ChatGPT brings to the table.In this guide, we’ll explore how ChatGPT, a conversational AI developed by OpenAI, is revolutionizing the way we interact with technology. From its basic features to its more advanced capabilities, and even its limitations, you’ll discover how to make ChatGPT work for you—whether you’re using it for personal projects or professional tasks.
Read More
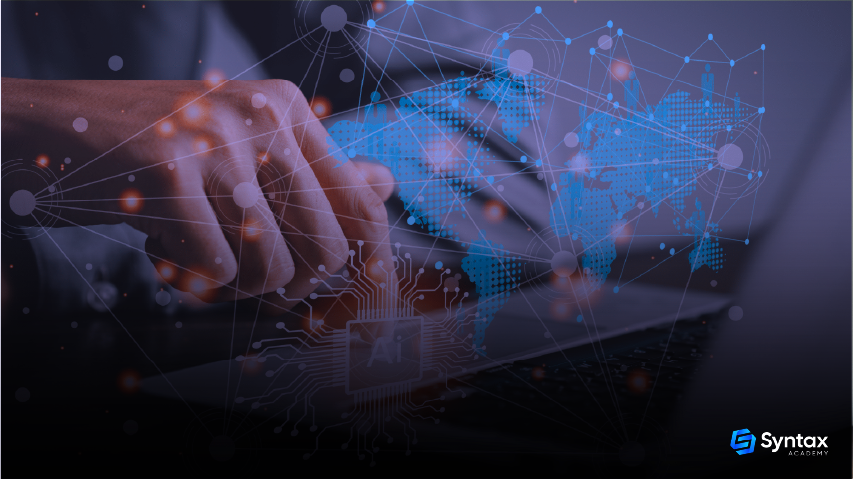
Career & Jobs
How to Boost Your Tech Career: Tips for Skill Development and Networking
By: Martha James
Feeling stuck in your tech career? You’re not alone. The tech world moves faster than we can keep up with sometimes, and if you don’t stay ahead, you might find yourself falling behind.Picture this: you're a developer who just landed a fantastic job, but a few years later, those skills that once made you shine are now seen as the bare minimum. So, what’s the game plan? Whether you're just getting started or aiming to level up, staying relevant is all about two things: building your skills and growing your network.In this blog, we’ll explore practical strategies to keep your tech career thriving. From participating in hackathons to contributing to open-source projects and developing meaningful connections, these tips will help you stay ahead in the fast-moving tech industry.
Read More
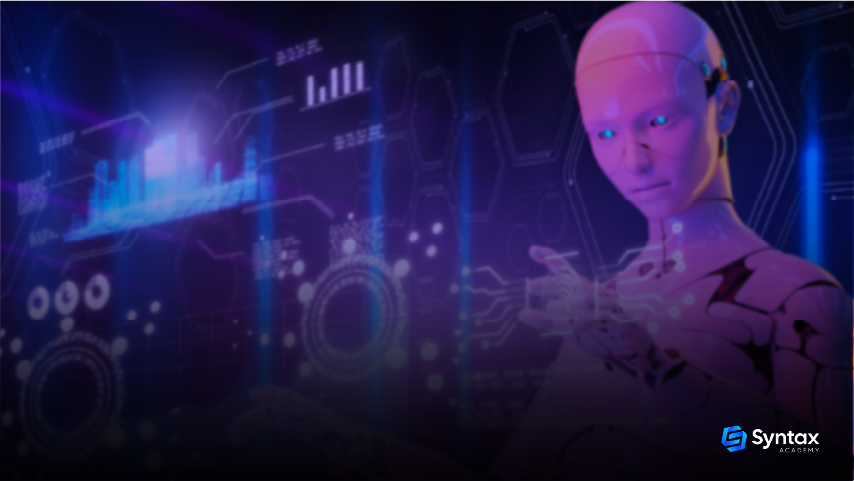
BI & Analytics
Excel for Business Intelligence: Unlocking the Power of Data Analysis
By: Martha James
Feeling stuck in your tech career? You’re not alone. The tech world moves faster than we can keep up with sometimes, and if you don’t stay ahead, you might find yourself falling behind.Picture this: you're a developer who just landed a fantastic job, but a few years later, those skills that once made you shine are now seen as the bare minimum. So, what’s the game plan? Whether you're just getting started or aiming to level up, staying relevant is all about two things: building your skills and growing your network.In this blog, we’ll explore practical strategies to keep your tech career thriving. From participating in hackathons to contributing to open-source projects and developing meaningful connections, these tips will help you stay ahead in the fast-moving tech industry.
Read More
Professionals from Industry Pioneers Choose
Syntax Academy for Skill Mastery
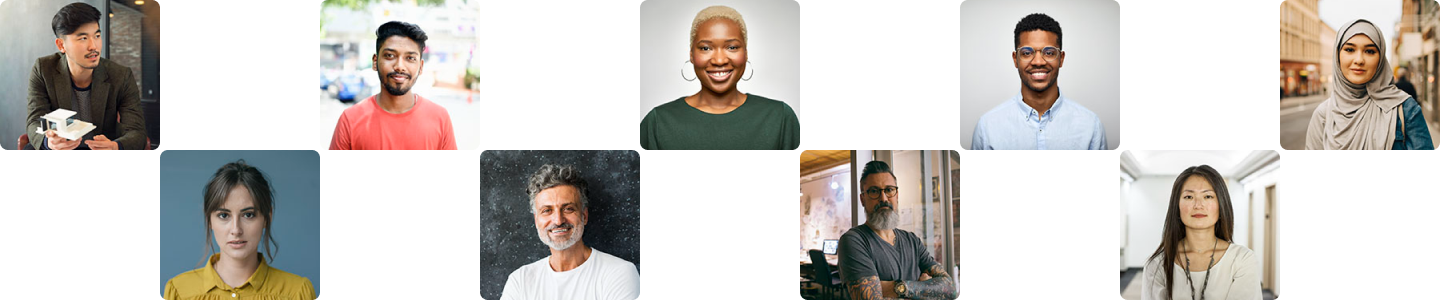